shared knowledge
shared progress
We create software to support decision
making on chemical safety
We create software to support decision making on chemical safety
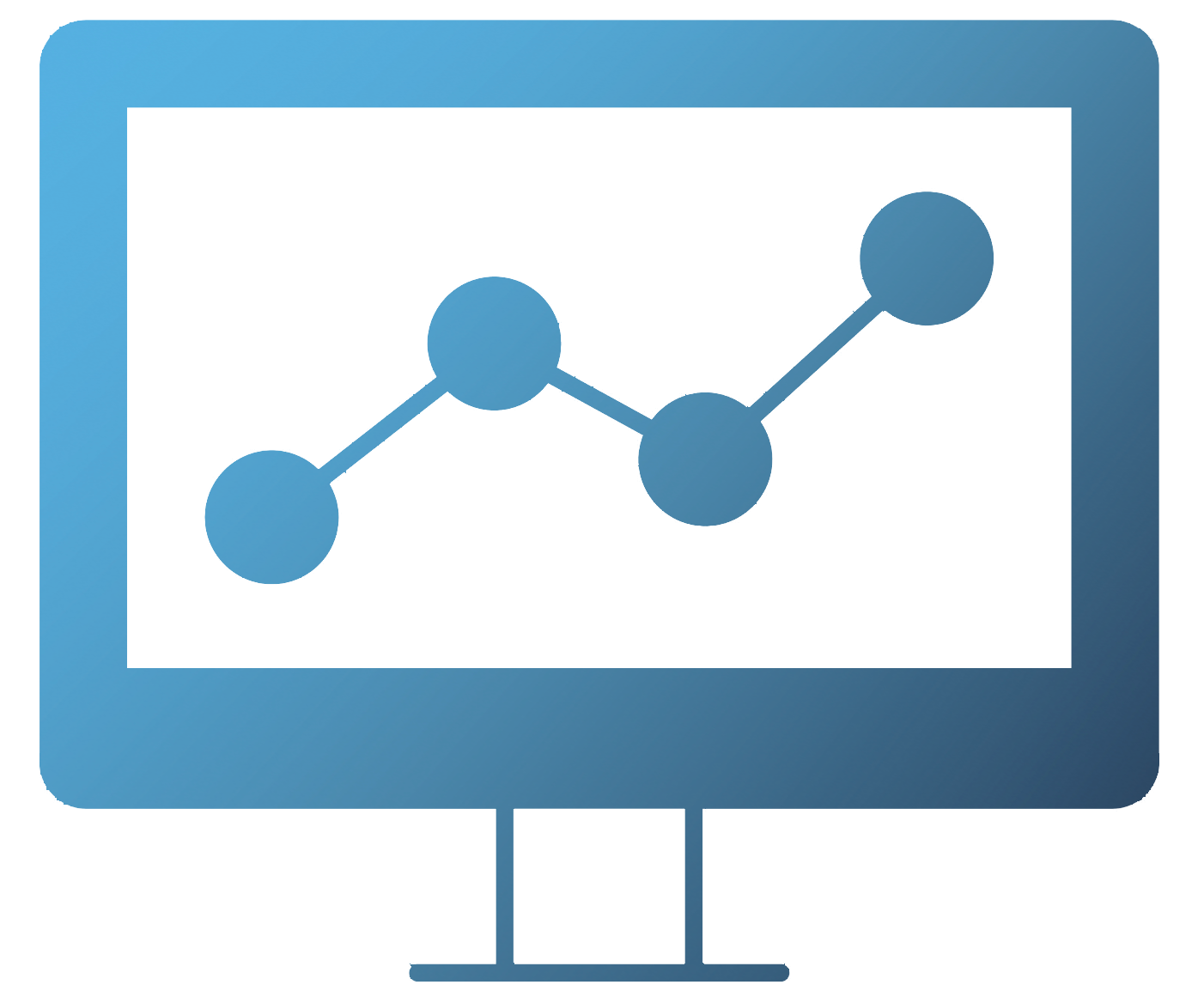
- Solutions
In silico software solutions to support your chemical safety assessments
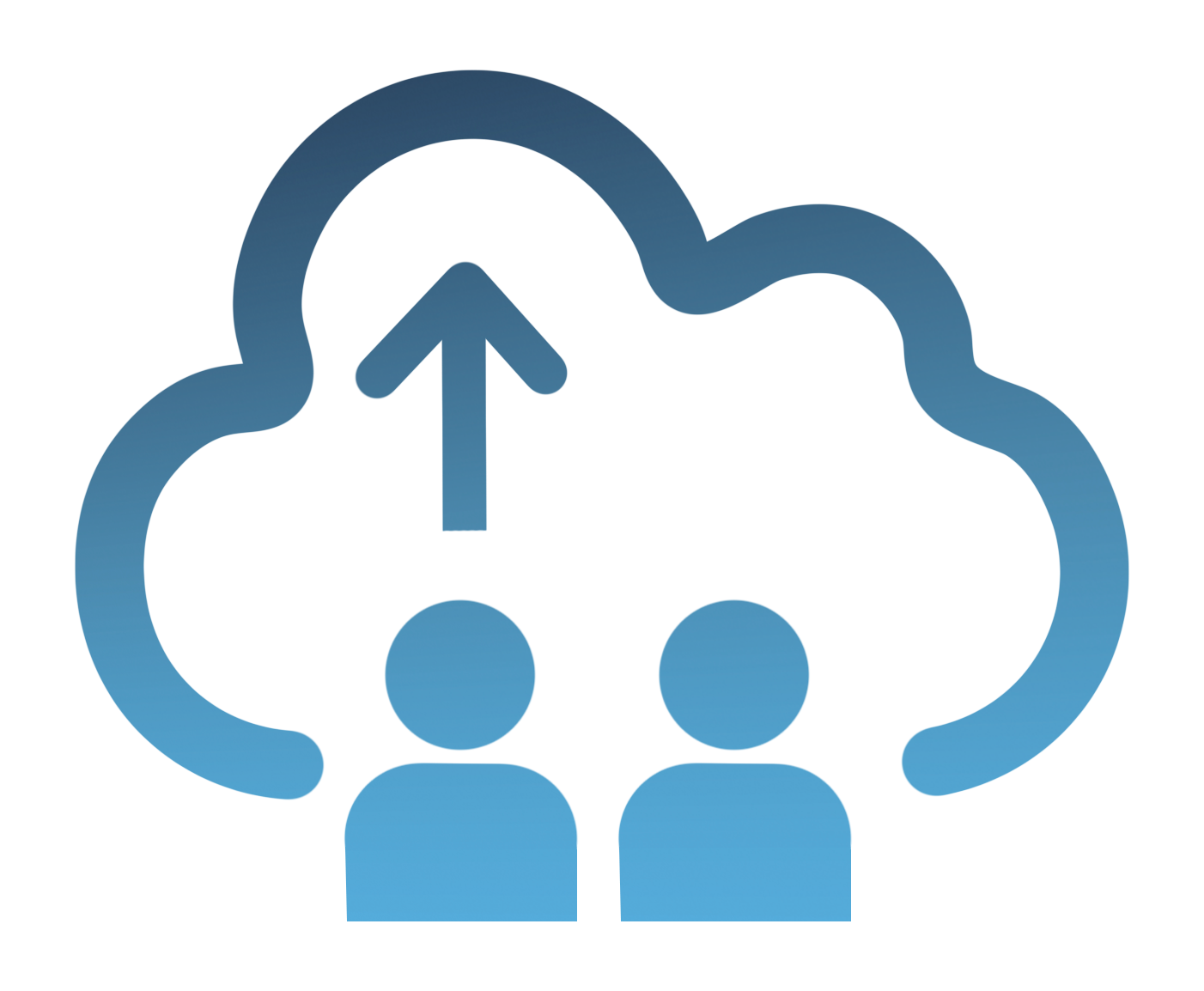
- Data sharing
Precompetitive data sharing initiatives that support your risk assessments by providing access to high-quality proprietary data
Our technology is designed by scientists, for scientists, in collaboration with industry stakeholders and regulators
Our people
We achieve more when we work together
Driven by our purpose; to enable informed decision making on chemical safety, our team of talented individuals often work in solution teams, combining a diverse set of skills to support the development of our world-class scientific software solutions that align with member and regulatory expectations.
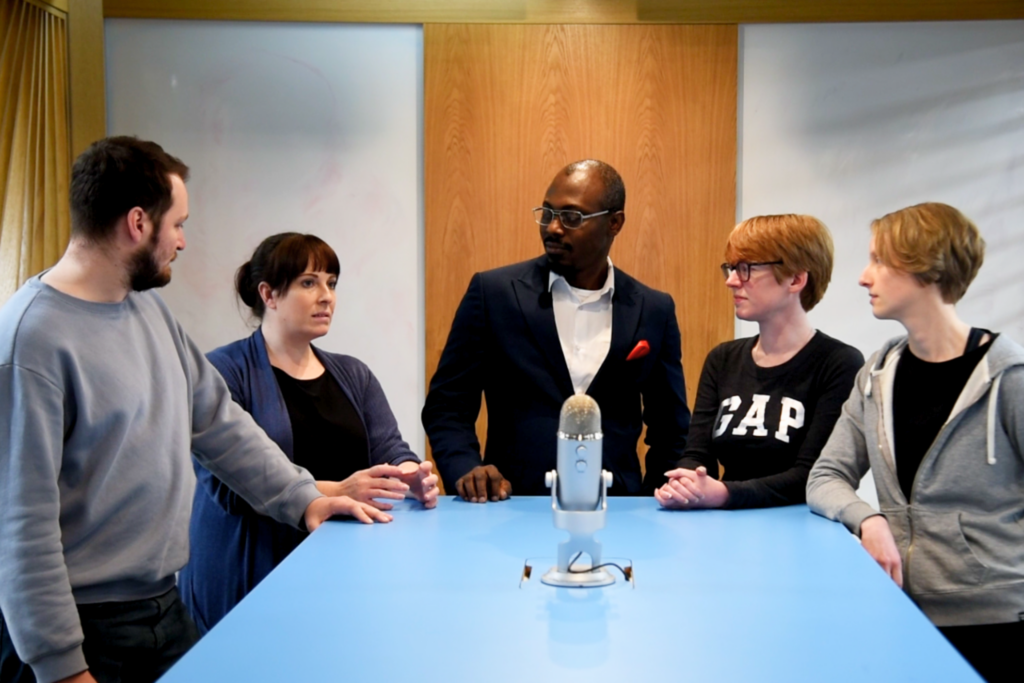
Our values
We create an environment where everyone can succeed
At Lhasa, we believe that it is important to foster a culture of diversity and inclusion. We are committed to creating an environment where our colleagues feel comfortable to be themselves and feel empowered. By encouraging and embracing diversity and inclusion, we experience better discussions, decisions, and outcomes.
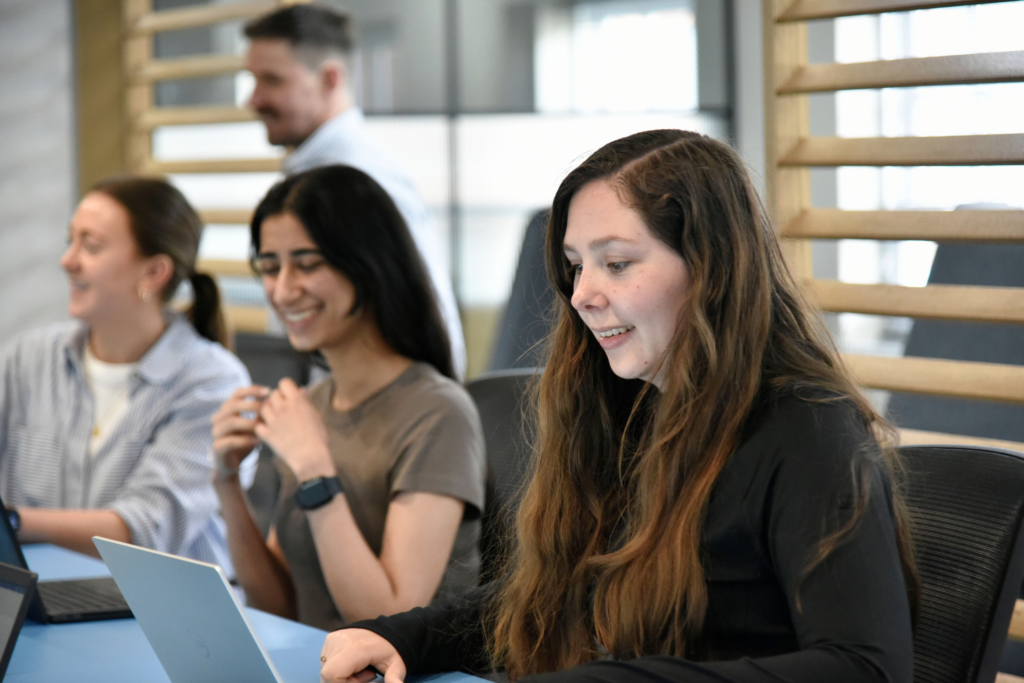