Safety profiling in drug discovery
Supporting more comprehensive safety profiling decision making
It takes a huge amount of time (estimated 10-15 years) and money (estimated US$2.6 billion) to bring one drug candidate to market following its discovery. There is also a low success rate – only 0.02% of drugs in development make it to market. Given the low success rate, if drug candidates are going to fail, they need to fail fast and fail cheaply. We are passionate about supporting safety assessors in making complex decisions by working with our members to accelerate the drug development process.
Our solution
It is widely reported that toxicology is the biggest driver of compound attrition during drug discovery. We can support your toxicology safety assessments – by helping you to generate enhanced safety profiles and supporting your decision making using our representation of relevant knowledge – within our in silico software solutions; Kaptis and Derek Nexus.
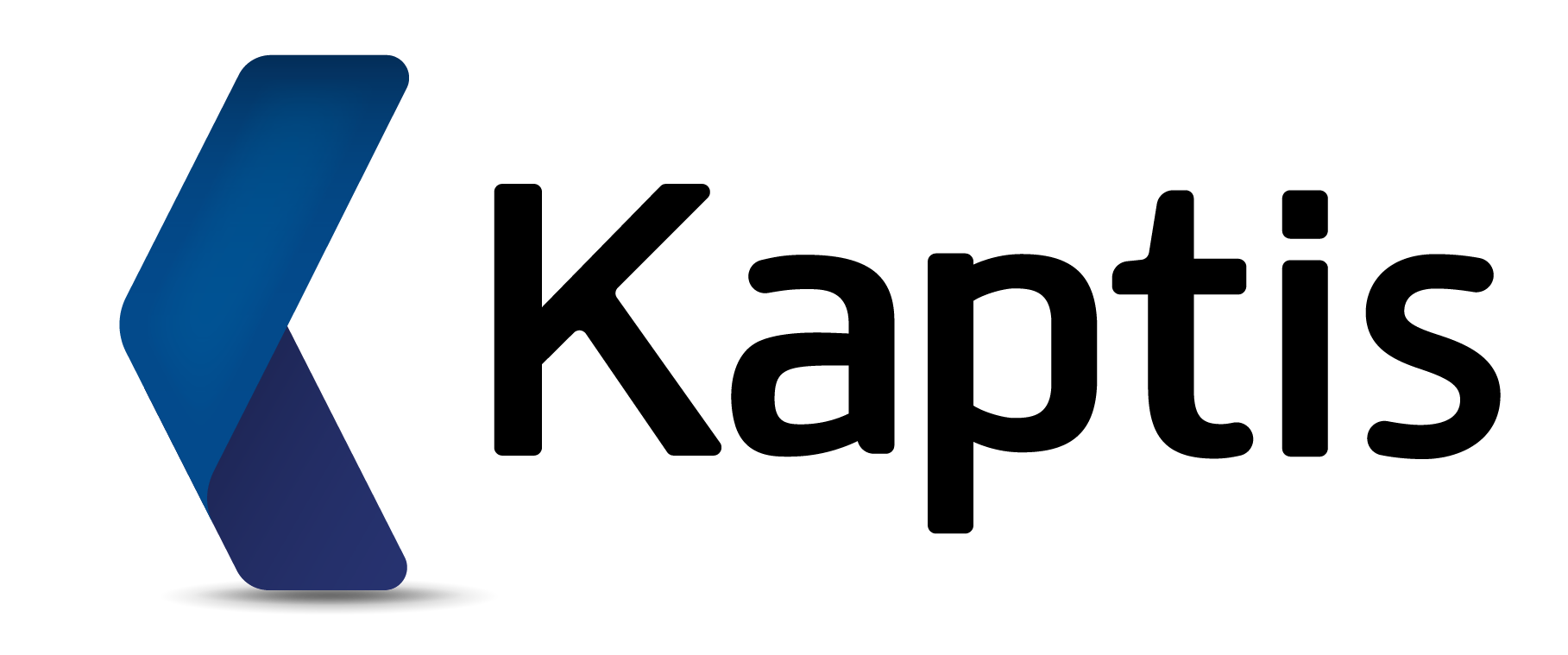
Kaptis is a novel software solution which can help you to profile your compounds in relation to the risk of human adverse events, using adverse outcome pathways (AOPs) to organise knowledge.
Kaptis highlights
Explore relevant knowledge, assay data and predictions
Our Kaptis AOP knowledge framework – which has been created, curated and peer reviewed by expert Lhasa scientists – can help you to explore relevant knowledge, assay data and predictions.
Understand the toxicological liabilities of your lead compounds
Combine existing knowledge and your own assay data in a meaningful way, using adverse outcome pathways (AOPs) within Kaptis. This integrated approach to testing and assessment (IATA) can help you to quickly understand the toxicological liabilities of your lead compound.
Improve the efficiency of your early testing strategy
Kaptis helps you to answer the question; “What should I do next?” – more specifically, which assay to run next and which adverse outcome (AO) to be concerned about. Resulting in more informed, faster, and more cost-effective human safety assessment decisions.
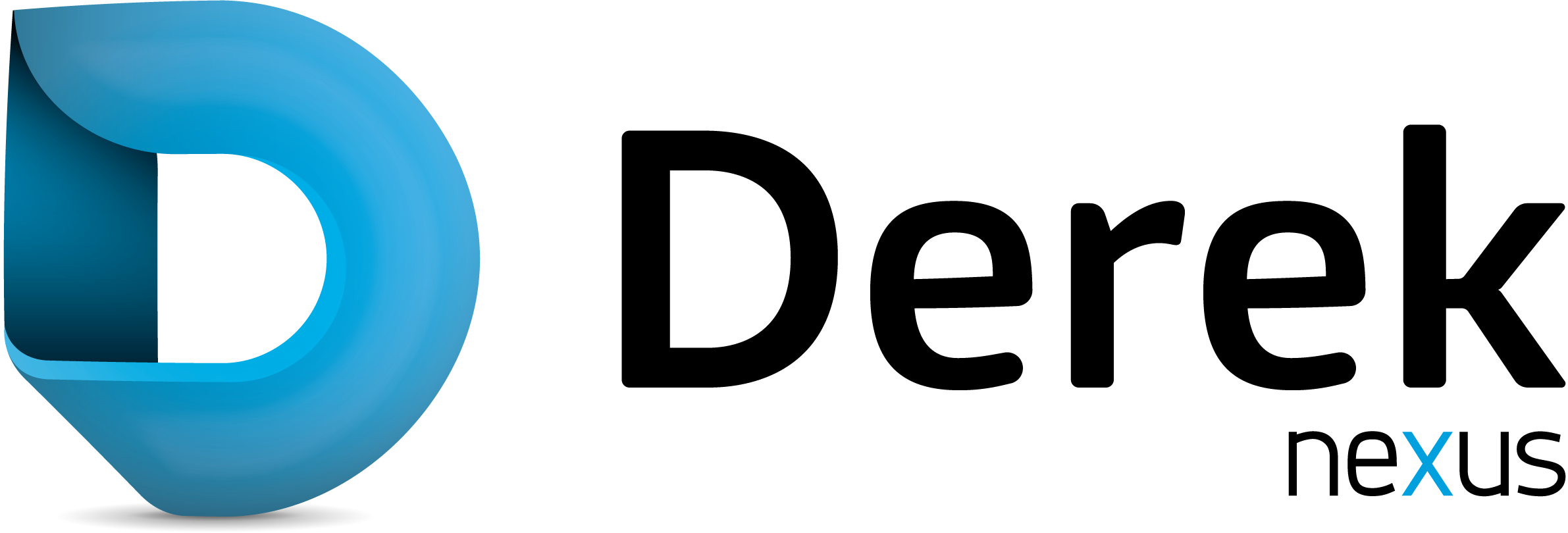
Derek Nexus is an expert, knowledge-based prediction software that can help you to profile your compounds against key toxicity endpoints using expert-derived toxicophores, based on both public and proprietary data.
Derek Nexus highlights
Triage your compound library
Identify toxicophores highlighting possible hazard quickly, through expert-curated structure-activity relationships (SARs) in your virtual screens.
Access expert-curated scientific knowledge
Utilise the scientific knowledge within Derek Nexus which is derived from both public and proprietary member data sources. We update Derek Nexus as new data becomes available.
Build confident assessments
Use the transparent predictions, knowledge and supporting evidence in Derek Nexus to build more confident assessments. Understand the SARs behind predictions from scientific comments within Derek Nexus – which describe the mechanism of action – and follow up on the knowledge to gather key supporting pieces of evidence within the supporting references.
Regulatory support
Using Kaptis and Derek Nexus within your drug discovery phase can enhance regulatory submissions through the provision of more comprehensive safety assessments.
Supporting information
What is an AOP?
An approach to documenting causal relationships between biological processes which lead to adverse outcomes/toxicity. AOPs start with a molecular initiating event (MIE) and through additional key events (KEs), lead to an adverse outcome (AO). Each sequential KE (including the MIE and AO) are connected to each other through key event relationships (KERs). Each KE should be measurable and therefore can be linked to a relevant assay. This framework enables data to be contextualised and organised in an intuitive way, to support decisions with respect to chemical safety assessments.
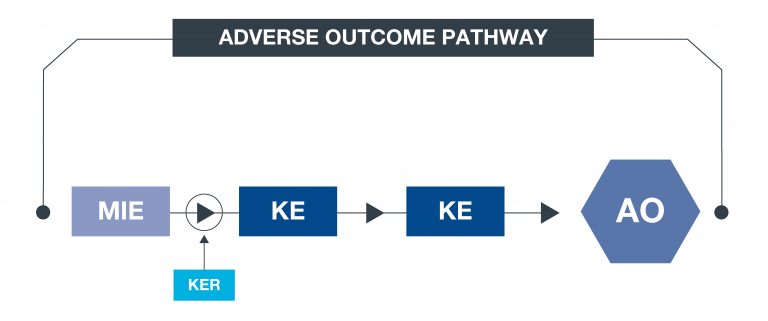
Related publications
Poster
- Mar 2024
- Carcinogenicity assessment, In silico mutagenicity assessment, Safety profiling in drug discovery
Poster
- Mar 2024
- Carcinogenicity assessment, In silico mutagenicity assessment, Safety profiling in drug discovery
Paper
- Oct 2023
- Safety profiling in drug discovery
Recent blogs
Diversity serves as a catalyst for innovation at Lhasa. We believe in cultivating a workplace culture that encourages collaboration, and empowers every …
Following the success of the 2022 Science of Stability (SOS) Conference in Philadelphia, we’re excited to announce its return to Barcelona! The …
We are delighted to share our important new open access article: In silico prediction of pharmaceutical degradation pathways: A benchmarking study using …