Skin sensitisation assessment
Evaluating the skin sensitisation potential and potency of chemicals
Historically, the skin sensitisation potential and potency of substances have been mainly assessed by in vivo studies on animals.
Various regulations and guidelines have introduced measures to encourage the phasing out of animal testing, this has prompted the development and use of non-animal methods to identify potential skin sensitisers – chemical substances that cause an allergic reaction following skin contact.
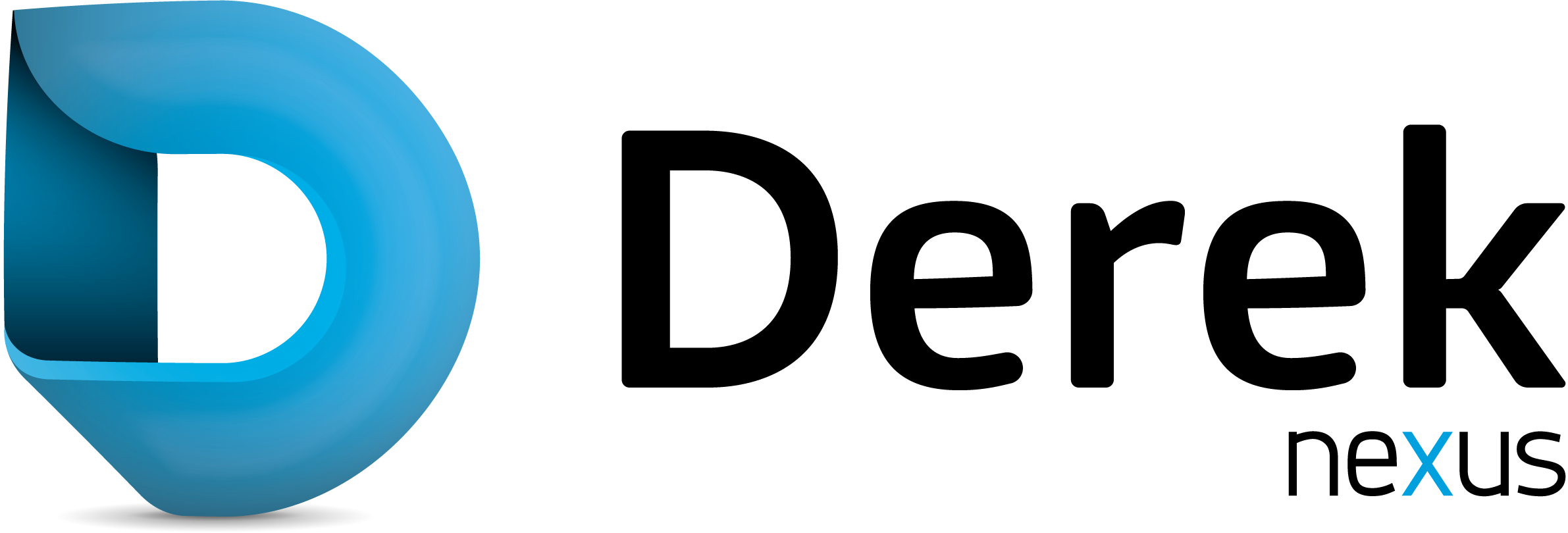
Derek Nexus contains well-established Structure-Activity Relationship (SAR) knowledge, which can help you to assess the skin sensitisation potential and potency of chemicals.
Derek Nexus highlights
Access potency predictions and negative predictions
Extrapolating hazard identification to risk, Derek Nexus provides EC3 predictions to inform potency. Negative predictions allow confidence when classifying a compound as a non-sensitiser, when query compounds do not fire any skin sensitisation alerts.
Identify strong/extreme sensitisers of particular concern
High Potency Category (HPC) alerts consist of a set of structural features within a mechanistic domain which are likely to be associated with extreme skin sensitisation potential in the Local Lymph Node Assay (LLNA). The presence or absence of alerts for skin sensitisation HPC provides information about which of the Dermal Sensitisation Thresholds (DSTs) is the most appropriate to use.
Use as part of a defined approach
The use of Derek Nexus with in vitro assays and in chemico assays can predict the skin sensitisation potential of chemicals. One of the three approved defined approaches mentioned within OECD Guideline No.497, ITSv1 (Integrated Testing Strategy version 1) by Kao, includes Derek Nexus as a required information source.
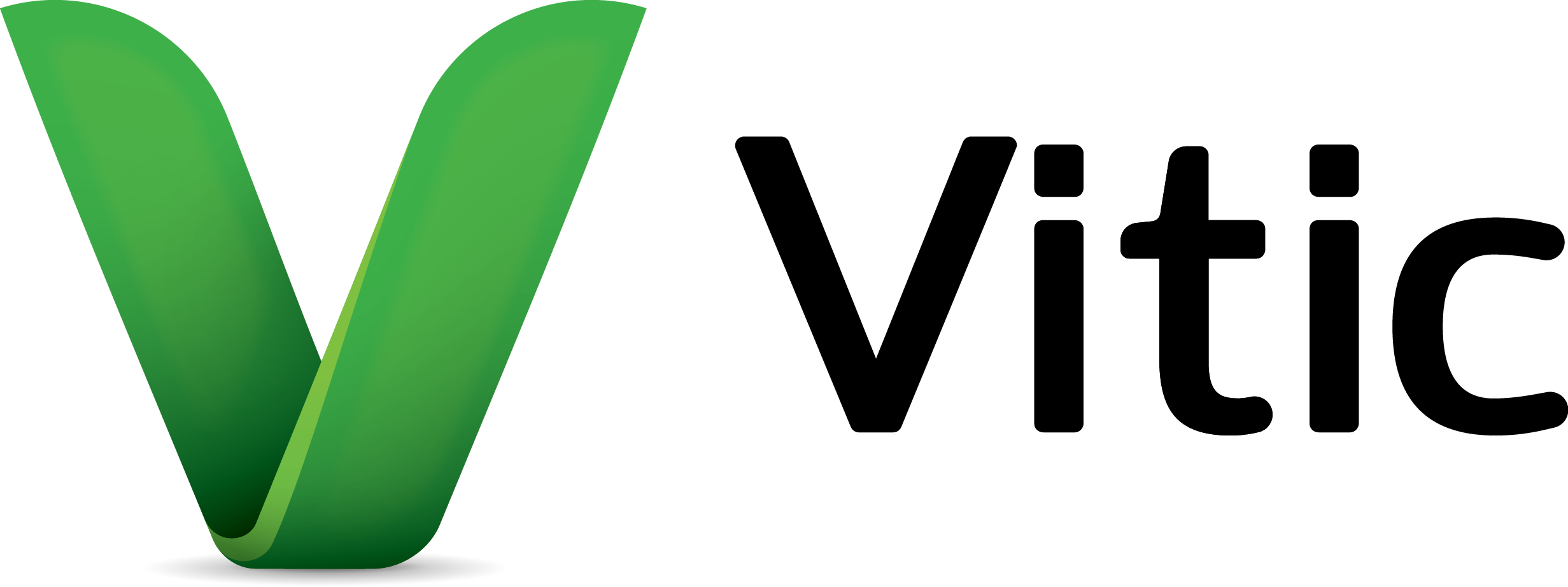
Vitic provides high-quality, peer-reviewed skin sensitisation data, which can support your individual safety assessments and read-across approaches.
Vitic highlights
Explore high-quality supporting data
Access both in vivo skin sensitisation data and data from New Approach Methodologies (such as in chemico /in vitro assays etc.).
Improve read-across assessments
With new data added on a regular basis, users of Vitic can access current skin sensitisation data to support predictions and read-across assessments.
Access information derived from a range of sources
Contains expert curated, high-quality, peer-reviewed data from both published and unpublished sources.
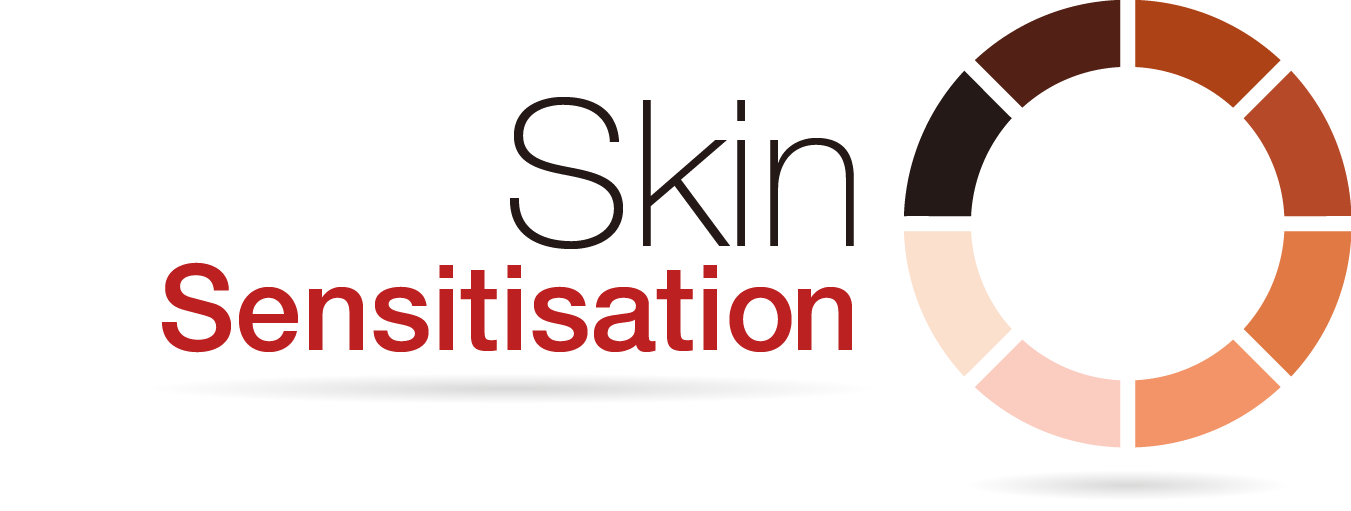
Skin Sensitisation Defined Approach ITSv1 provides hazard and potency skin sensitisation predictions in place of using animal tests, which can assist your hazard characterisation and risk assessment.
Defined Approach ITSv1 highlights
Use Derek as part of the ITSv1 defined approach
OECD Guideline No.497 recommends the use of a combination of non-animal methods to predict whether a chemical can cause skin allergies, known as a defined approach (DA). Quickly and efficiently predict hazard and potency categories by combining a Derek Nexus prediction with h-CLAT and DPRA data.
Generate accurate predictions quickly
The defined approach has a high level of accuracy compared to animal and human data.
Automate your Data Integration Procedure (DIP)
Our ITSv1 web application considers applicability domains, the handling of missing information sources and assessment of the prediction confidence.
Regulatory support
The prediction of skin sensitisation is a key requirement for a number of chemical safety assessments and regulations:
Registration, Evaluation, Authorisation & Restriction of Chemicals (REACH)
REACH contains several specific measures and general provisions designed to establish and enforce the principle that animal testing should be performed only as a last resort. Derek Nexus, Vitic and ITSv1 provide an alternative method to carrying out in vivo studies on animals.
Classification, Labelling and Packaging (CLP)
CLP Regulation states that all hazardous chemicals (substances and mixtures) placed on the market must be classified, labelled and packaged according to the CLP Regulation, displaying the correct hazard for skin corrosion/irritation. As such, Derek Nexus, Skin sensitisation Defined Approach ITSv1 and Vitic offer skin sensitisation predictions which can be used for chemical safety assessment.
OECD Guideline No. 497
Defined Approaches on Skin Sensitisation can be used to meet other regulations such as REACH and CLP. The guideline recommends using defined approaches to predict whether a chemical can cause skin allergies. Our ITSv1 tool is a step towards full replacement of animal testing for skin sensitisation and employs the 3Rs principle - the replacement, reduction, and refinement of animals used for product testing and scientific research.
EU regulation 1223/2009
EU regulation 1223/2009 prohibits the use of animal testing for cosmetic ingredients. The use of Derek Nexus as part of a Defined Approach can deliver predictions that have a high concordance with the LLNA assay and provides a significant step forward in assessing skin sensitisation.
ICH Q3E
The upcoming ICH Q3E guideline will recognise the need to assess the sensitisation potential of extractable and leachable (E&L) compounds. Expert knowledge within Derek Nexus can be used to predict the sensitisation potential of Extractables and Leachables.
Related publications
Paper
- Feb 2025
- Skin sensitisation assessment
Poster
- Mar 2024
- Skin sensitisation assessment
Recent blogs
In the ever-evolving landscape of pharmaceutical regulations, staying abreast of the latest guidelines is crucial for ensuring the safety and efficacy of …
A new paper, “Data-driven federated learning in drug discovery with knowledge distillation”, was recently published in Nature Machine Intelligence, the leading journal …
At Lhasa we know that there are many challenges involved in carrying out risk assessments for drug substances and drug products, to …