In silico mutagenicity assessment
Streamlining the computational assessment and
control of mutagenic impurities
In silico methods are routinely used for the assessment of the mutagenic potential of chemicals under several regulatory regimes. The ICH M7 guideline provides a practical framework that can be applied to the identification, categorisation, qualification and control of potentially genotoxic impurities to limit potential carcinogenic risk. The use of two complementary (qualitative) structure activity relationship [(Q)SAR] prediction methodologies is required; one expert rule-based and the other statistical-based. To identify the mutagenic potential of drug impurities for regulatory purposes, these (Q)SAR prediction methodologies can be used as a substitute for the bacterial reverse mutation assay (Ames test).
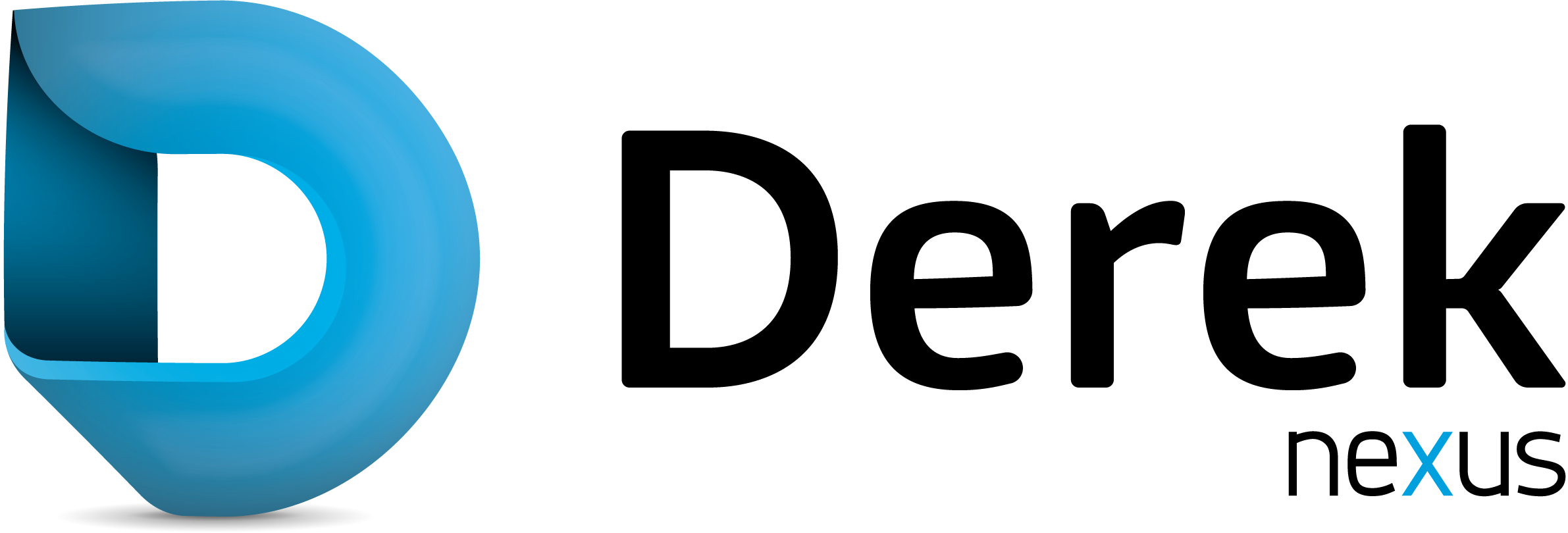
Derek Nexus is an expert-knowledge based software, which can help you to meet ICH M7 by providing fast and accurate toxicity predictions.
Derek Nexus highlights
Access over 40 years of expert structure-activity relationship (SAR) knowledge for the mutagenicity endpoint
Derek Nexus contains well-established Structure-Activity Relationship (SAR) knowledge for the mutagenicity endpoint. The SAR knowledge is derived from both public and proprietary data sources and is regularly updated as new data becomes available.
Retrieve negative predictions for bacterial in vitro mutagenicity
Streamline the expert review process with negative predictions for bacterial in vitro mutagenicity. Derek Nexus gives an interpretable method to determine the reliability of a negative prediction when assessing impurities under the ICH M7 guideline.
Simplify expert review for submission under ICH M7 guideline
Transparent, scientifically robust predictions, supported by high-quality curated data with extensive coverage of chemical space permits rapid expert review. Derek Nexus can be used in tandem with Sarah Nexus and Vitic to assign an ICH M7 classification for impurities of an Active Pharmaceutical Ingredient (API).
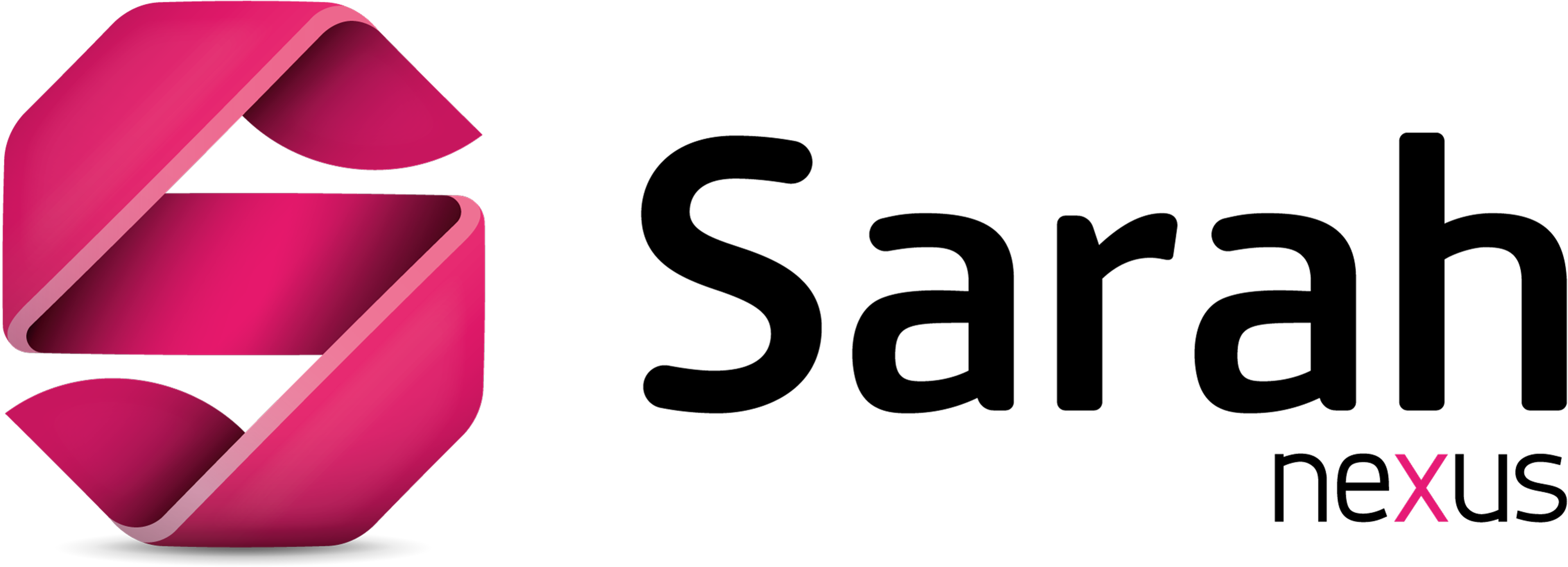
Sarah Nexus is a statistical-based software which can help you to meet ICH M7 by identifying potentially toxic chemicals through the prediction of mutagenicity.
Sarah Nexus highlights
Predict for bacterial mutagenicity
Employ a unique, machine-learning methodology to predict the bacterial mutagenicity of a query compound.
Apply Sarah Nexus as part of an ICH M7 workflow
Combining a Sarah Nexus and Derek Nexus prediction provides you with the means to meet the computational toxicological assessment requirements of the ICH M7 guidelines.
Utilise donated Ames mutagenicity data
In addition to published data, the Sarah Nexus training set uses Ames mutagenicity data donated by Lhasa members to produce a self-organising hierarchical network (SOHN) model containing hundreds of hypotheses, covering a vast area of chemical space.
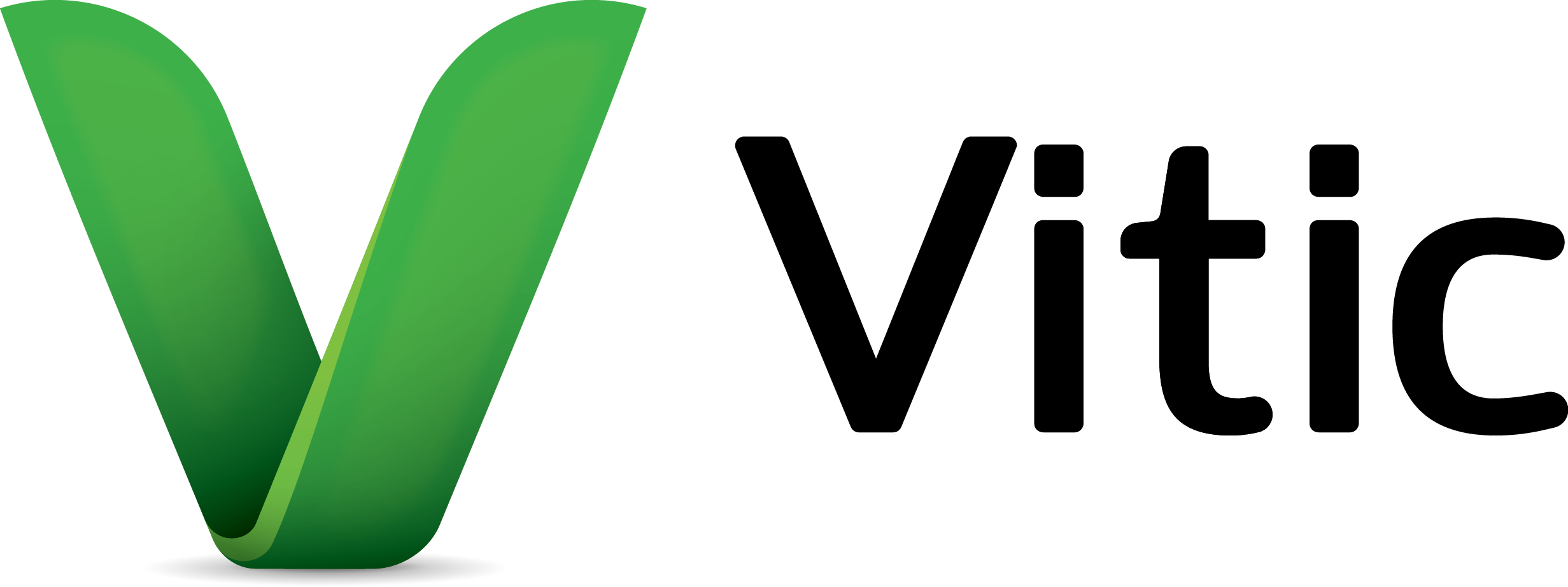
Vitic is a structure-searchable toxicity database and data management system. When used alongside Derek Nexus and Sarah Nexus, Vitic can help you to retrieve existing data available for your compound.
Vitic highlights
Access expertly curated and peer reviewed mutagenicity and carcinogenicity data
Our regularly updated toxicity database offers high-quality, Lhasa-curated data. To maintain accuracy between Vitic data and the original data source, data is extracted manually by our scientists and peer reviewed by a second member of the science team.
A fast and efficient searching experience
Rapidly find relevant supporting examples for your impurities by structure, substructure or similarity searching, thereby adding weight to expert review.
Collaborate with industry experts through data sharing
Lhasa-hosted data sharing initiatives, including the aromatic amines, excipients and complex nitrosamines consortia, allow anonymous sharing of mutagenicity data. These consortia avoid the need for duplicate testing and allow members to exchange knowledge with other industry experts through recurrent consortium meetings.
Regulatory support
ICH M7
The ICH M7 guideline states that “Two (Q)SAR prediction methodologies that complement each other should be applied. One methodology should be expert rule-based & the second should be statistical-based”. Combining a Derek Nexus expert rule-based prediction and a Sarah Nexus statistical prediction can provide a full ICH M7 classification.
ISO 10993
ISO 10993 discusses the strategy for evaluating carcinogenicity of medical devices, materials and/or their extracts over a period of the major portion of lifespan of the in vivo test. To ensure animal welfare and minimize the animal testing, Derek Nexus and Sarah Nexus can be used to evaluate the carcinogenicity of medical devices.
Guidance on the establishment of the residue definition for dietary risk assessment
The aim of the EFSA guidance is to guide the complex process of identifying the pertinent residue components that should be considered for dietary risk assessments of chemical active substances. Our (Q)SAR tools, Derek Nexus and Sarah Nexus, can be used for metabolite hazard identification and characterisation of these compounds.
Related publications
Poster
- Jul 2024
- Carcinogenicity assessment, In silico mutagenicity assessment
Recent blogs
In the ever-evolving landscape of pharmaceutical regulations, staying abreast of the latest guidelines is crucial for ensuring the safety and efficacy of …
A new paper, “Data-driven federated learning in drug discovery with knowledge distillation”, was recently published in Nature Machine Intelligence, the leading journal …
At Lhasa we know that there are many challenges involved in carrying out risk assessments for drug substances and drug products, to …