Our people
Our work is only possible by recruiting, developing, and retaining world-leading talent!
We are driven by our purpose; To enable informed decision making on chemical safety. In line with our purpose, we provide support to our members through data, knowledge, and science that helps to build more confident risk assessments, whilst relying less on animal testing.
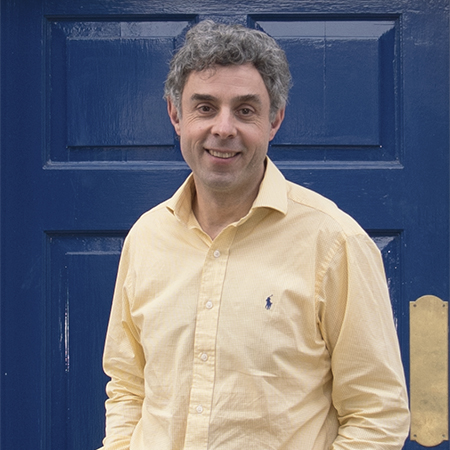
Dr Chris Barber
Chief Executive Officer
In our pursuit, we are led by Dr Chris Barber as our Chief Executive Officer, and inspired by the needs of our members. We invest in research to develop our science, collaborate to share knowledge and data, and create intuitive software to make this accessible.
Dr Crina Heghes
Director of Applied Sciences
The Applied Sciences team focuses on understanding our members’ needs and supporting the application of our solutions to solve real-world problems. This team is led by Dr Crina Heghes. Our Applied Sciences team works collaboratively with members, future members, and regulators to ensure that our solutions support good decisions which align with regulatory expectations.
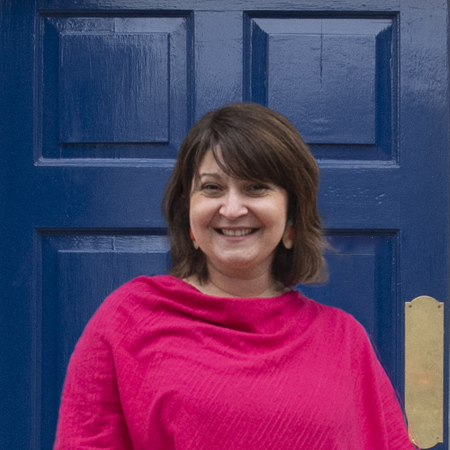
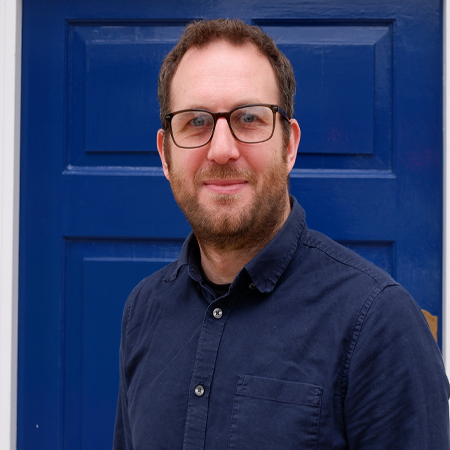
Dr Adrian Fowkes
Director of Science
The Science team is led by Dr Adrian Fowkes and focuses on developing the science behind our solutions. Our expert scientists provide the scientific insight needed to allow our members to make more confident decisions on chemical safety using our solutions.
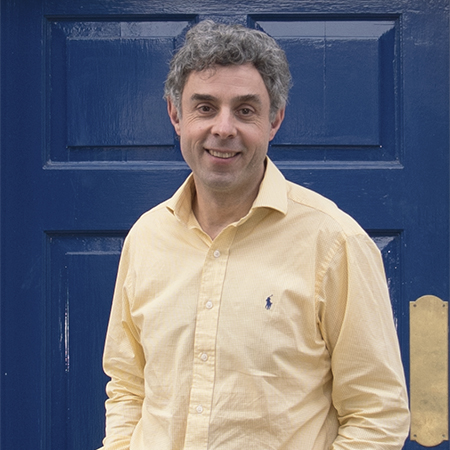
Dr Chris Barber
Chief Executive Officer
In our pursuit, we are led by Dr Chris Barber as our Chief Executive Officer, and inspired by the needs of our members. We invest in research to develop our science, collaborate to share knowledge and data, and create intuitive software to make this accessible.
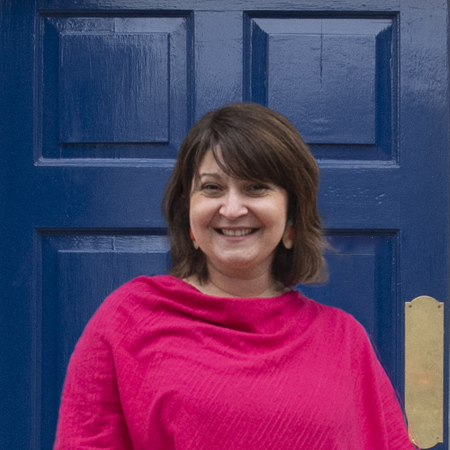
Dr Crina Heghes
Director of Applied Sciences
The Applied Sciences team focuses on understanding our members’ needs and supporting the application of our solutions to solve real-world problems. This team is led by Dr Crina Heghes. Our Applied Sciences team works collaboratively with members, future members, and regulators to ensure that our solutions support good decisions which align with regulatory expectations.
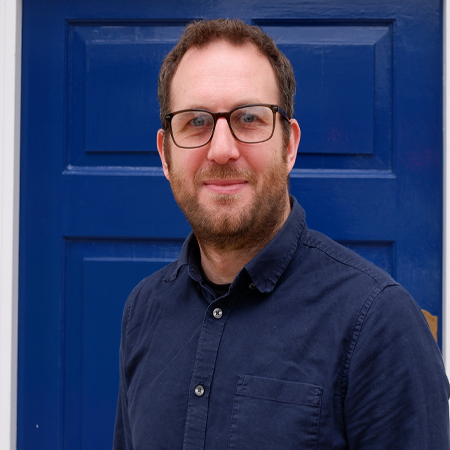
Dr Adrian Fowkes
Director of Science
The Science team is led by Dr Adrian Fowkes and focuses on developing the science behind our solutions. Our expert scientists provide the scientific insight needed to allow our members to make more confident decisions on chemical safety using our solutions.
Our solutions make scientific progress a reality for members around the world, this is made possible by our dedicated team of Architects, Delivery Managers, Software Developers, Software Testers and Solution Owners.
Our valuable Lhasa support functions enable us to deliver our innovative software solutions and include IT, Finance, Business Improvement, People and Culture, and Facilities.